Embodied AI
Embodied AI: Artificial intelligence that leaves the digital world to inhabit the physical world
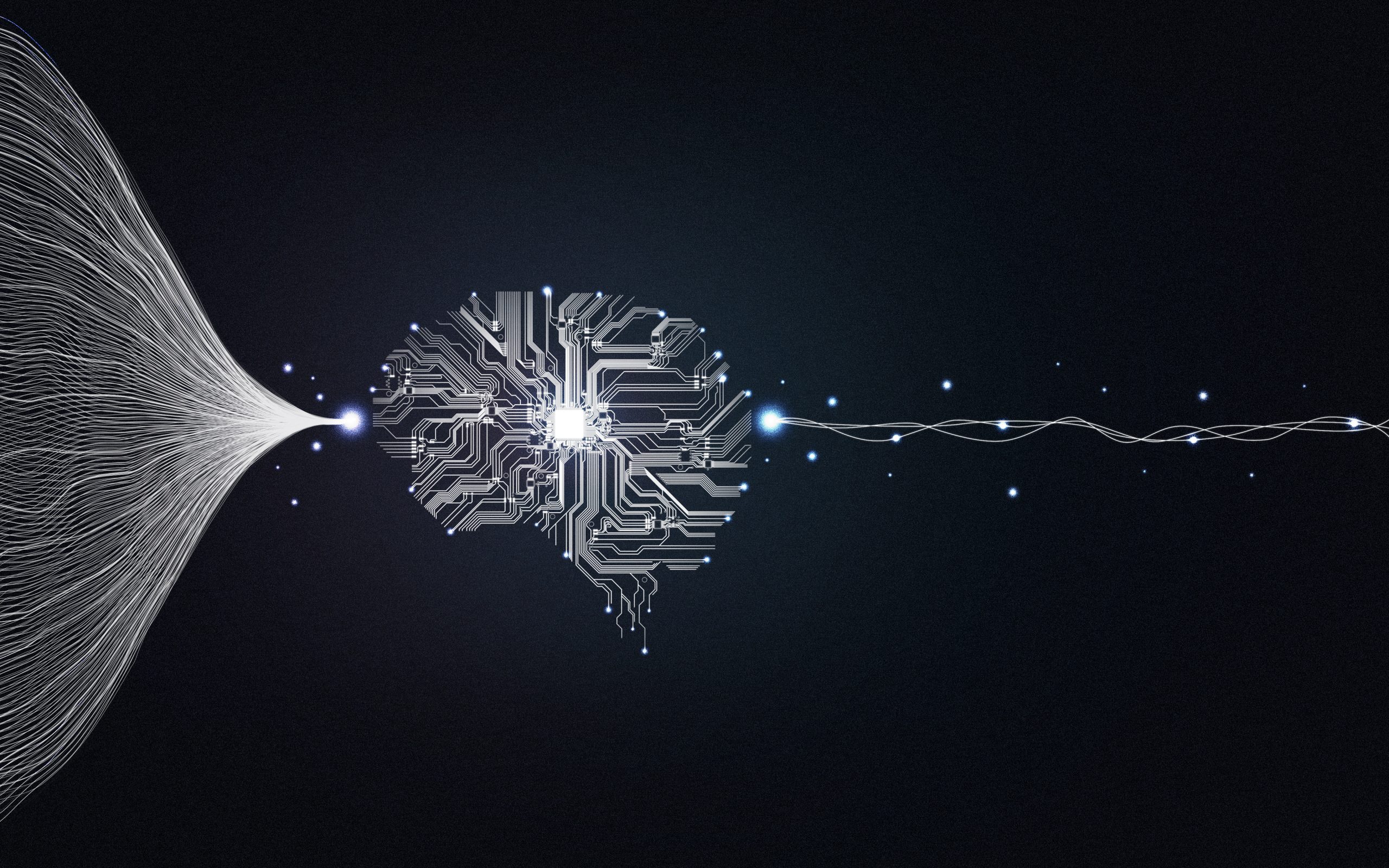
Jeremy Kahn looks at the evolution of physical AI, from generalist models to autonomous robots and drones, in a world where technology increasingly interacts with the real environment
This article has been translated using artificial intelligence
Artificial intelligence is no longer just software living in the cloud: it is now taking physical form, interacting with the real world and changing the way we work, move and live. Its evolution has taken a crucial step: now, AI not only analyzes information, but interacts with the physical world. This transformation is redefining the relationship between humans and machines, driving advances in sectors such as healthcare, mobility, industry and logistics.
The Future Trends Forum of the Bankinter Foundation of Innovation has delved into this revolution, bringing together international experts to explore its technological, economic and social impact. The result of this analysis will be a soon-to-be-published report that will serve as a roadmap for understanding the challenges and opportunities of AI in the physical world.
The series of articles that we start today anticipates some of the keys to this report, beginning with the vision of one of the experts who participated in the forum; Jeremy Kahn, Editor of Artificial Intelligence at Fortune Magazine and author of the book Mastering AI: A Survival Guide to Our Superpowered Future. With a global view on the evolution of AI, Kahn has closely followed the development of physical AI and its potential to transform key industries. With him, we explore the historical challenges and recent advances that are shaping the future of physical AI, from the development of more generalist models to the evolution of autonomy in robots, drones and vehicles.
To watch Jeremy Kahn’s presentation, you can do so in this video:
Physical AI’s cycles of hype and stagnation
Artificial intelligence has evolved through cycles of excitement and disappointment, and the history of physical AI is no exception. In 2005, the DARPA Grand Challenge generated great expectations for autonomous vehicles, with the belief that within a decade they would be on every road. However, the reality has been much more complex. Full autonomy remains a challenge and adoption is proving slower than anticipated.
In 2016, attention shifted to generative AI with the success of DeepMind and AlphaGo, as well as with the appearance of the paper Attention Is All You Need in 2017, which laid the groundwork for transformers. For years, physical AI has been on the back burner as the world focused on language models like ChatGPT.
Now, according to Kahn, physical AI is making a strong comeback thanks to new strategies in its development.
The paradigm shift in physical AI: from multiple models to generalist models
Historically, physical AI operated with a combination of specific models. Each of these models served an independent function: perception, decision making and mapping. This approach, while effective in controlled environments, made integration and scalability difficult. In recent years, however, the trend has shifted toward unified models, capable of combining multiple capabilities in a single neural network.
World models and their impact
One of the most significant advances is the implementation of world models. These models seek to replicate an internal representation of the environment that allows AI systems to understand and act more efficiently. Rather than separating perception and decision, the idea is to merge them into a single model trained to interpret the world and make decisions based on that understanding.
Prominent examples include:
- Wayve with its Gaia model, applied to autonomous cars.
- World Labs, founded in 2024 by renowned artificial intelligence researcher Fei-Fei Li, which seeks to develop 3D world models and as of today is already a unicorn startup.
The interest in these models is no accident; with the development of these unified models, physical AI could reach a tipping point.
But the key to making these models truly effective is not just in the representation of the environment, but in how they can be translated to different applications without having to start from scratch. This is where foundational models in robotics come into play.
Foundational models in robotics: the “GPT” of robots
The evolution towards foundational models in robotics follows a similar path to that of Large Language Models (LLMs) in language processing. Just as LLMs have revolutionized text generation, foundational models in robotics seek to provide generalist capabilities that can be applied to multiple types of robots without additional training.
These foundational models benefit directly from advances in world models. While a world model allows an AI system to build a global understanding of the environment, a foundational model uses that knowledge to act in different scenarios and with different types of hardware. In other words, world models provide insight and context, while foundational models provide the capability for flexible and generalist execution.
Companies such as Physical Intelligence and Covariant are developing models capable of operating different robots with minimal customization. This approach would allow physical AI systems to be deployed in different environments without specific programming, reducing costs and accelerating adoption. The combination of world models and foundational models promises a future where robots will be able to quickly adapt to new environments and tasks without the need for extensive training, bringing us closer to a turning point in the evolution of physical AI.
Autonomous drones: the most radical breakthrough in physical AI
Another rapidly evolving field is autonomous drones, one of the most advanced applications of physical artificial intelligence. Traditionally dependent on human operators or GPS, these devices are reaching an unprecedented level of independence, with key implications in both the military and commercial arenas.
In the war in Ukraine, drones with increasing autonomy have marked a milestone in the application of AI in warfare, redefining strategies on the battlefield. In other scenarios, such as Israel, models have been developed that maintain human intervention in final decision making to avoid critical errors. This development raises a debate about the ethical and operational limits of AI in high-risk environments.
Outside the military, the potential of autonomous drones is being deployed in key sectors such as underwater exploration, rescue and natural resource exploitation. Companies in a variety of sectors are using these devices to map oceans, discover shipwrecks and perform oil rig inspections with unprecedented efficiency. These innovations are driven by the need to overcome dependence on GPS and improve navigation based on advanced sensors and machine vision.
The advancement of autonomous drones is a clear example of how physical artificial intelligence is transforming industries and redefining machine capabilities. Their development will continue to shape the future of strategic sectors, from security and logistics to the exploration of unexplored environments.
Where does the human fit into physical AI?
Kahn raises a key question: does physical AI need a body of its own or can it inhabit the human body? The answer is not simple, but technology is moving in directions that defy this dichotomy. Today, there are devices that turn the human itself into the AI interface.
Smart glasses with embedded AI, such as the Ray-Ban Meta, are designed to enhance perception of the environment and provide real-time assistance. Wearable devices with generative AI have also emerged, such as AI Pins, which act as advanced personal assistants without the need for screens. Even more disruptive are brain-computer interfaces, which allow devices to be controlled by thought, a further step towards the fusion of humans and technology.
This concept of “Embodied AI” therefore encompasses not only autonomous robots, but also systems where the human body becomes the physical platform for artificial intelligence. As these technologies advance, the boundary between biology and technology becomes increasingly blurred, redefining the role of the human in the evolution of physical AI.
Is it the “ChatGPT moment” for physical AI?
Kahn posits that generative AI experienced its big moment in 2022 with ChatGPT, but physical AI has yet to have its “media explosion.”
Some signs indicate that moment may be near:
- OpenAI, after abandoning robotics in 2019, has restarted its physical AI program.
- Companies like NVIDIA and Tesla are investing massively in robot world models.
- The use of natural language will make it easier to interact with robots, reducing the need for specialized programming.
In the next few years, we could see a “ChatGPT moment” for robots, with more generalist and versatile models.
In short, the evolution of physical AI is accelerating thanks to three major trends:
- From separate models to generalist models.
- Drones and autonomous systems with greater independence
- More natural human-machine interfaces
Kahn makes it clear that the advancement of physical AI is a transformation in the way humanity interacts with technology.
The big question is not whether physical AI will change the way we interact with the world, it is to what extent do we want to delegate critical tasks and decisions to increasingly autonomous machines?
Are we ready to share our space with an intelligence that, in addition to processing data, also acts?